Predicting the existence of heavy nuclei using machine learning
A collaboration between the Facility for Rare Isotope Beams (FRIB) and the Department of Statistics and Probability (STT) at Michigan State University (MSU) estimated the boundaries of nuclear existence by applying statistical analysis to nuclear models, and assessed the impact of current and future FRIB experiments.
More than 99.9 percent of the visible universe is made from 286 stable isotopes. However, the nuclear force allows many more unstable, radioactive isotopes to exist. That instability often comes from how difficult it is to keep cohesion when there are many more neutrons than protons in a given nucleus. We may never observe most of these unstable isotopes, but these short-lived inhabitants of the nuclear borderlands matter: they govern the processes in stars that create all the stuff around us, and what we are made of.
Over a year ago, FRIB and STT at MSU formed a new collaboration between nuclear physics and the statistical sciences. This collaboration, led by the joint hire of statistics researcher Dr. Léo Neufcourt, was born to get nuclear physics and statistics to work together on building predictive models that will answer fundamental questions about rare isotopes.
In light of the recent discovery of eight new rare isotopes of the elements phosphorus, sulfur, chlorine, argon, potassium, scandium, and calcium (the heaviest isotopes of these elements ever found), the FRIB/STT team estimated the boundaries of nuclear existence in the calcium region with a full quantification of uncertainties, assessing the impact of the experimental discovery on nuclear structure research. The work is published in Physical Review Letters.
The group used a statistical framework called Bayesian machine learning, where statistical model parameters and predictions are obtained in the form of a posterior probability. In essence, this framework allows for using new data (evidence) to estimate how probable certain related outcomes are. The methodology they employ is explained in a joint paper in Physical Review C. After an individual analysis of several nuclear models, their predictions are combined using Bayesian weights based on the ability of each model to account for the most recent discoveries.
Using the latest mass data and evidence of existence of chlorine, argon and sulfur along with what is currently known about existing nuclei, the researchers applied a Bayesian approach with nuclear theory models to predict what new heavy nuclei might be, and with what probability they might exist. This analysis is a form of what is sometimes known as supervised machine learning. The algorithm is first given nuclear models and information on experimentally found nuclei. It explores a myriad of possibilities but then concentrates around the most relevant ones considering the current experimental data. The methodology allows researchers to quantify their predictions' uncertainties precisely and reliably.
In that matter, they estimate that heavier calcium isotopes, up to calcium-70, could exist (see figure). According to these results, calcium-68 for instance is 76 percent likely to exist. This estimate may change as scientists discover new isotopes in the same region, which the team will use to update its predictions. In the future, FRIB will allow scientists to potentially create calcium-68 or even calcium-70.
The team is working on several other uses of Bayesian machine learning with applications to nuclear physics, including a project to calibrate the particle beam in the FRIB accelerator. The methodology is expected to have direct applications to areas which need quantified data from model-based extrapolations, such as nuclear astrophysics.
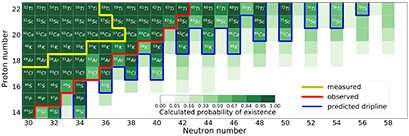
Read more at: https://phys.org/news/2019-02-heavy-nuclei-machine.html#jCp
Explore further: Researchers discover heaviest known calcium atom; eight new rare isotopes discovered in total
More information: Léo Neufcourt et al. Neutron Drip Line in the Ca Region from Bayesian Model Averaging, Physical Review Letters (2019). DOI: 10.1103/PhysRevLett.122.062502
Léo Neufcourt et al. Bayesian approach to model-based extrapolation of nuclear observables, Physical Review C (2018). DOI: 10.1103/PhysRevC.98.034318
Read more at: https://phys.org/news/2019-02-heavy-nuclei-machine.html#jCp